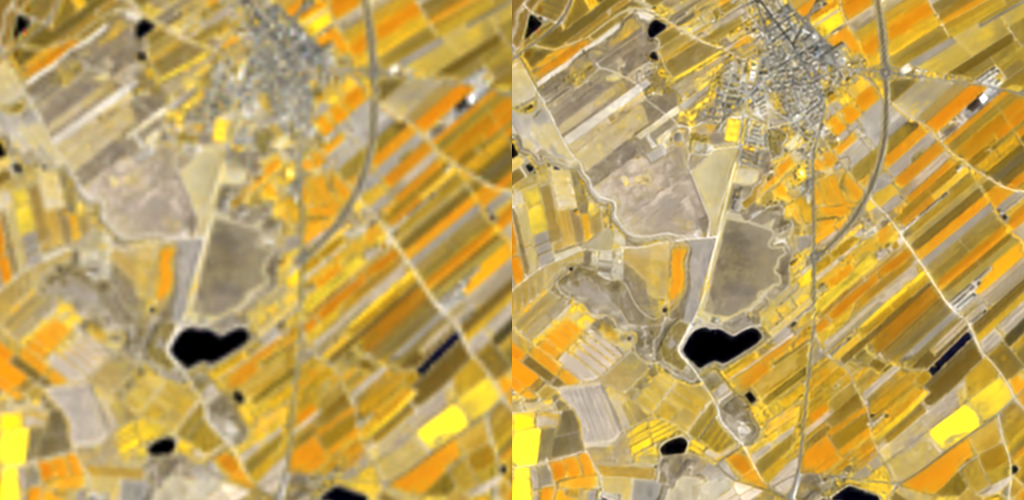
Sentinel-2 Enhance button: 5-meters resolution for 10 ban...
Enhance button is a very common movies trope, where a character scrolls through some video footage or photos and asks […]
Enhance button is a very common movies trope, where a character scrolls through some video footage or photos and asks […]
=> Several data access centres are being renovated at CNES, ESA, and their first versions often lack some of the […]
SWOT, the Surface Water and Ocean Topography mission was successfully launched more than a year ago (Dec 16, 2022). Hydrologists […]
Iota2 is constantly evolving, as you can check at the gitlab repository. Bugs fix, documentation updates and dependency version upgrade […]
The results presented here are based on published work : V. Bellet, M. Fauvel, J. Inglada and J. Michel, « End-to-end […]
=> Update the 8th of January, 2024 : A new change in the interface from the Copernicus Dataspace on January […]
=> The results presented here are based on work published in the journal paper: F. Mouret, D. Morin, H. Martin, […]
The iconic Khumbu Glacier in Nepal is fed by several tributaries, such as this branch in the Lingtren–Khumbutse catchment. Recently […]
=> As the resolution of our satellite missions improve, the data volume of output products increases, and the share of […]
Three years ago, we started the phase-0 study of a mission named Sentinel-HR : S-HR would be a satellite mission to […]